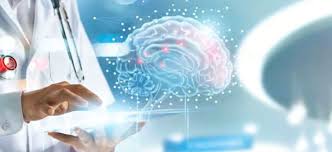
Risk Categorization
The FDA stated that the AI/ML-based SaMD (Artificial Intelligence / Machine Learning-based Software as a Medical Device) will follow the same risk categorization of SaMD.
International Medical Device Regulator Forum’s (IMDRF) risk framework of SaMD has two major factors to provide information about the intended use of SaMD.
These two major factors are:
1) The Significance of information provided by the SaMD to the healthcare decision, which identifies the intended use of the information provided by the SaMD – i.e., to treat or diagnose; to drive clinical management; or to inform clinical management
2) State of healthcare situation or condition, which identifies the intended user, disease or condition, and the population for the SaMD – i.e., critical; serious; or non-serious healthcare situations or conditions.
After evaluating the software according to the above two factors, the AI/ML-based SaMD will be placed into one of the four (I-IV) categories.
(Figure 1 retrieved from International Medical Device Regulator Forum, IMDRF)
*I signifies the lowest risk and IV is the highest risk.
*The figure can be used to reflect the risk associated with the clinical situation and device use.
Total Product Life Cycle (TPLC)
In order to maximize the potential of AI/ML devices, the FDA will use the TPLC approach that will balance safety and effectiveness.
The TPLC approach will allow the evaluation of premarket development, postmarked performance, and the organization itself to ensure continual excellence.
The general principle of this approach is to:
- Establish clear expectations on quality systems and good ML practices (GMLP);
- Conduct premarket review for those SaMD that require premarket submission to demonstrate a reasonable assurance of safety and effectiveness and establish clear expectations for manufacturers of AI/ML-based SaMD to continually manage patient risks throughout the lifecycle
- Expect manufacturers to monitor the AI/ML device and incorporate a risk management approach and other approaches outlined in “Deciding When to Submit a 510(k) for a Software Change to an Existing Device Guidance in development, validation, and execution of the algorithm changes (SaMD Pre-Specifications and Algorithm Change Protocol)
- Enable increased transparency to users and FDA using post-market real-world performance reporting for maintaining continued assurance of safety and effectiveness.
These four principles can be more generalized as:
1. Quality systems and good machine learning practice.
2. Initial premarket assurance of safety and effectiveness.
3. Approach for modifications after initial review with an established SPS and ACP
4. Transparency and real-time monitoring of AI/ML-based SaMD
The FDA has already cleared or approved several AI/ML-based SaMD with the locked algorithm.
Its performance is hard to predict since it can only be assessed after examining how the software evolves through the consumption of real-world experience over time.
As a result, the intended output may be different than what it was originally given clearance for.
The FDA will continually revise the temporary regulatory framework of this powerful tool in order to provide safe and effective guidelines to developers, manufacturers, and patients.
You can read more about the full proposed regulatory framework published by the FDA here.
More about FDA: https://www.fda.gov/home