The new article addresses the aspects related to various matters associated with the licensing process for establishment licenses.
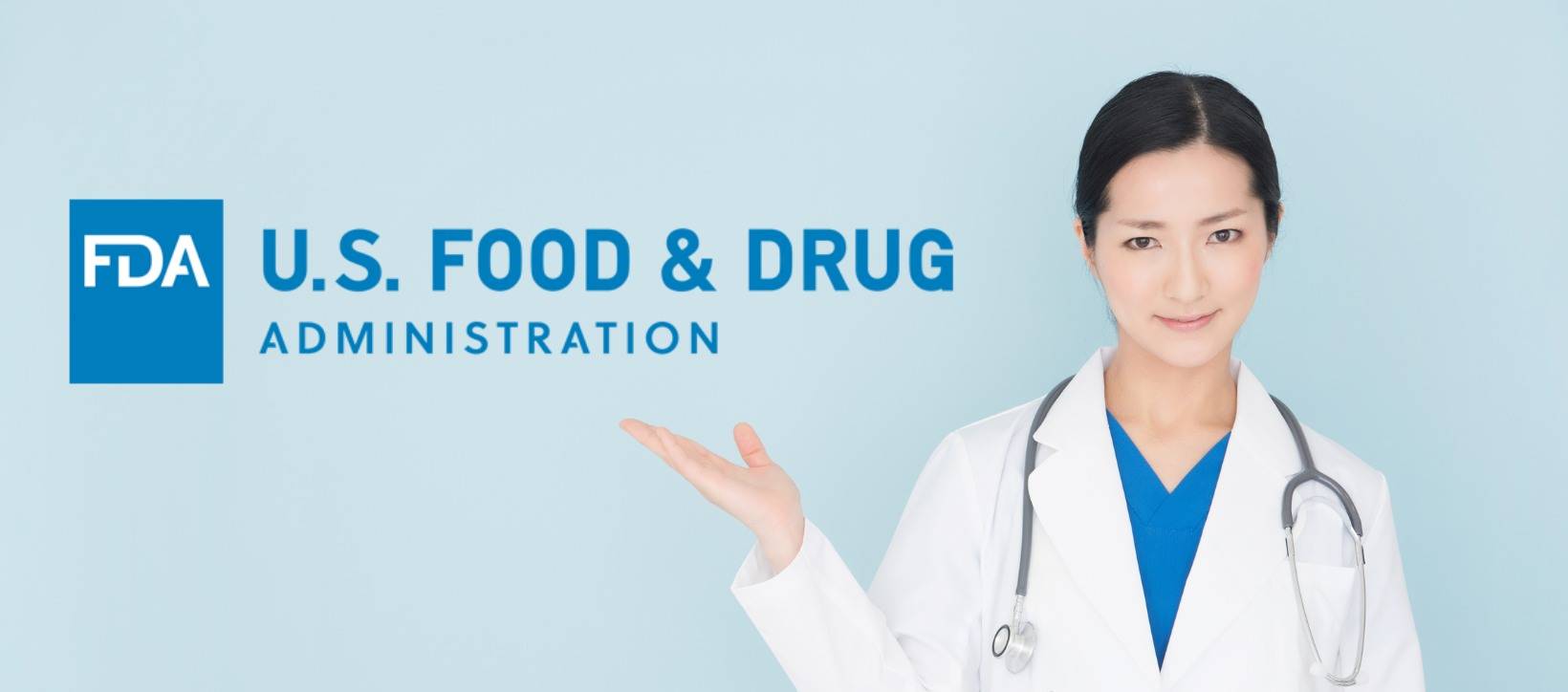
Table of content
The Food and Drug Administration (FDA or the Agency), the US regulating authority in the sphere of healthcare products, has published a guidance document dedicated to the approach to be followed when assessing the credibility of computational modelling and simulation in medical device submissions.
The document provides an overview of the applicable regulatory requirements, as well as additional clarifications and recommendations to be taken into consideration by medical device manufacturers and other parties involved to ensure compliance thereto.
At the same time, provisions of the guidance are non-binding in their legal nature, nor are they intended to introduce new rules or impose new obligations.
Moreover, the authority explicitly states that an alternative approach could be applied, provided such an approach is in line with the existing legislation and has been agreed with the authority in advance.
The document highlights, inter alia, certain special considerations related to specific categories of credibility evidence.
Code Verification Results
According to the guidance, in the framework’s fifth step, it is highly recommended to use the credibility factors for code verification as defined in ASME V&V 40.
This is especially important in the context of medical device software, where a clear distinction must be made between software verification and model verification/validation. As explained further by the FDA, these two processes, while both crucial, differ in their scope and definitions.
Software verification may encompass code verification of the computational model, but typically, the latter is treated separately, necessitating consideration of the specific Context of Use (COU).
In this respect, the authority further refers to the guidance titled “Guidance for the Content of Premarket Submissions for Software Contained in Medical Devices” for appropriate testing and reporting recommendations.
In cases where computational models are not part of the device, such as in silico device testing or clinical trials, their code verification is distinct from device software verification/validation.
If a commercial software package is used in developing these models, it’s recommended to refer to the software manufacturer’s information on software quality assurance and code verification.
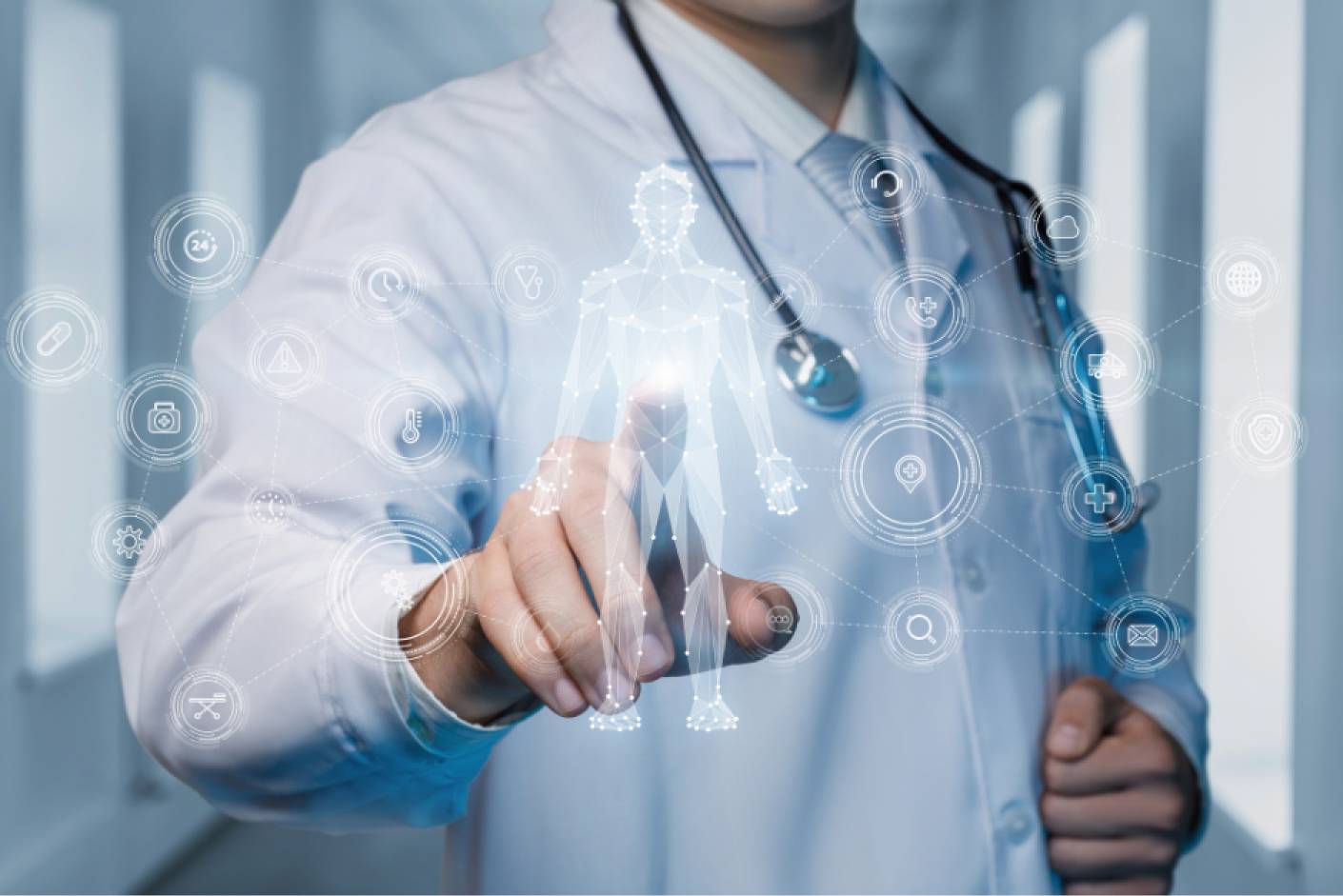
Model Calibration Evidence
For the fifth step in the framework, there’s a need to define credibility factors that address the goodness of fit, the quality of the comparator data, and the relevance of calibration activities to the COU.
It is also important to avoid conflating calibration evidence with validation evidence, ensuring that calibration data is distinct and not inclusive of data used for validation. The final values of all calibrated parameters that hold physical or physiological significance should fall within the expected ranges.
Apart from that, quantifying the “goodness of fit” is also recommended. When reporting calibration results, details such as the calibration procedure, calibrated parameters, prior distributions (if using a Bayesian approach), simulation details, experimental data, steps to prevent overfitting and numerical methods for obtaining results should be provided.
If calibration evidence serves as the primary source of credibility, a justification for the absence of model validation testing is needed, especially by referring to the assessed model risk.
In situations where no validation results are available, the relationship between calibration conditions and COU conditions, as well as calibration and COU quantities of interest, should be evaluated.
Bench Test Validation Results
Using the credibility factors defined in ASME V&V 40 is recommended for this category in the framework’s fifth step.
If the COU involves making in vivo predictions, the applicability of bench test validation results to the in vivo COU should be given special attention.
For prospectively planned validation, computational analysts performing simulations should be blinded to the bench test validation data to prevent potential bias.
In the case of validation against retrospective datasets, the applicability of validation results to the COU should be carefully considered, as the comparator data were not initially designed for validating the model for the current COU.
Similarly, for previously generated validation results, their applicability to the current COU requires careful assessment, including evaluating any differences and their impacts between the model used in the previous validation and the current model.
In Vivo Validation Results
In the framework’s fifth step, traditional validation evidence should use the credibility factors defined in ASME V&V 40.
However, it is important to mention that if the evidence takes another form, such as clinical trial results, it is recommended to generate and evaluate the evidence using the most appropriate best practices and methods.
This includes suitable statistical techniques, measures of sensitivity and specificity, and adhering to applicable regulatory requirements.
For prospectively planned validation, considering blinding the computational analysts to the validation data is advisable to mitigate bias.
Similar to bench test validation, the applicability of validation results to the COU is crucial, especially when using retrospective datasets.
Previously generated validation results also require careful consideration regarding their relevance to the current COU.
Population-Based Evidence
According to the document, evaluating population-based evidence involves a quantitative assessment of the closeness of the two populations by comparing means, variances, full distributions, or other appropriate statistical methods.
Relevant demographic information, anatomy, pathologies, and co-morbidities of the subjects used in the patient data, the clinical dataset used for validation, and the intended patient population should be provided and compared.
In cases where the evidence comes from a clinical study without subject-level data, generating and evaluating the evidence using appropriate best practices and statistical techniques is recommended.
Emergent Model Behavior
Emergent model behaviour is generally considered relatively weak evidence for model credibility compared to model validation. However, it can serve as useful secondary evidence.
The importance or relevance of the emergent behaviour to the COU should be evaluated, explaining why the model reproducing this behaviour instils confidence in the model for the COU.
Defining credibility factors for the relevance of the emergent behaviour to the COU, the sensitivity of emergent behaviour to model input uncertainty, and other factors is recommended for the framework’s fifth step.
Model Plausibility
Model plausibility, similar to emergent model behaviour, is generally a weaker argument for model credibility as it does not involve testing the model predictions directly.
If model plausibility evidence is the primary credibility evidence presented, a rationale for the absence of validation testing of the model should be provided, possibly by referring to the assessed model risk.
Evaluating how any assumptions impact predictions by comparing results using alternative model forms, preferably from higher-fidelity models, is important. Undertaking uncertainty quantification and sensitivity analysis for the model parameters is also crucial.
Calculation Verification/UQ Results Using COU Simulations
For calculation verification results, using the three calculation verification credibility factors defined in ASME V&V 40 is recommended for the framework’s fifth step.
Similarly, for UQ results, using the model input credibility factors defined in ASME V&V 40 is advised. If this type of evidence is generated, incorporating the calculation verification and/or UQ results when comparing COU predictions with any decision thresholds is important.
This should take into account the estimated numerical uncertainty and/or output uncertainty from UQ.
Conclusion
In summary, the present FDA guidance provides a detailed and descriptive overview of the considerations and recommendations for each category of credibility evidence within the framework of computational modeling. The document mostly focused on the aspects related to medical software.
How Can RegDesk Help?
RegDesk is a holistic Regulatory Information Management System that provides medical device and pharma companies with regulatory intelligence for over 120 markets worldwide. It can help you prepare and publish global applications, manage standards, run change assessments, and obtain real-time alerts on regulatory changes through a centralized platform. Our clients also have access to our network of over 4000 compliance experts worldwide to obtain verification on critical questions. Global expansion has never been this simple.